MLOps vs AIOps
in DevOps , AIOps , Machine Learning , MLOps
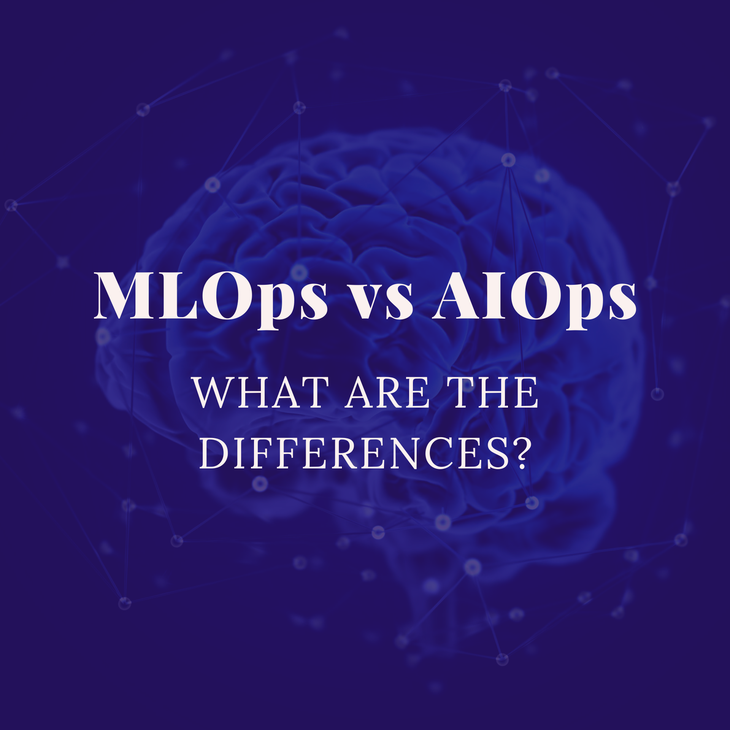
There is a tendency to confuse MLOps and AIOps. While there are some common characteristics between the two, MLOps and AIOps are two different domains, are applied differently, and serve different goals.
Let's start with the definitions.
What is AIOps?
AIOps, sometimes referred to as Artificial Intelligence for IT Operations or Algorithmic IT Operations is a term invented by Gartner in 2016 as an industry category for Machine Learning analytics technology that enhances IT operations analytics.
Gartner's official definition of AIOps is the following:
AIOps platforms utilize big data, modern machine learning and other advanced analytics technologies to directly and indirectly enhance IT operations (monitoring, automation and service desk) functions with proactive, personal and dynamic insight. AIOps platforms enable the concurrent use of multiple data sources, data collection methods, analytical (real-time and deep) technologies, and presentation technologies.Gartner, IncGartner, Inc, officially known as Gartner, is a global research and advisory firm providing information, advice, and tools for leaders in IT, finance, HR, customer service and support, communications, legal and compliance, marketing, sales, and supply chain functions. (source: wikipedia)
In other words, Machine Learning and Big Data are the pillars of AIOps.
AIOps' ultimate goal is enhancing IT operations. The central process in AIOps is the intelligent filtering of signals our of the noise in IT systems. We can understand the problem that AIOps solves as follow:
Logs, traces, tickets, incident data, system configuration status, and any information related to a given system's performance and operation are not intended to be the goal in itself, but rather the tools.
When the amount of collected data that can be examined and used to draw conclusions exceeds a certain limit, our understanding, assumptions, and priority patterns become distorted. Most importantly, identifying root causes and suggesting solutions become slower and less accurate.
AIOps is an approach to use machine intelligence to solve this problem by collecting, processing, and observing intelligently IT operations data in order to identify root causes and propose solutions fast. In some cases, AIOps have the capability to solve problems without human intervention.
What is MLOps?
Machine Learning Operations, or MLOps, is essentially a framework that focuses on the collaboration between data scientists and the operations unit within an organization.
If you are familiar with DevOps, you will not find MLOps hard to understand. DevOps is a practice for collaboration and communication between developers and operations professionals to help manage production, decrease the time-to-market, and implement a culture of continuous testing, development, and feedback. MLOps has almost the same definition, except that developers here are usually data scientists, AI specialists, and Machine Learning engineers.
In other words, MLOps follows a path similar to that of DevOps. While DevOps focuses on shortening the product life cycle by creating better products each time, MLOps drives insights that can be put into better use immediately.
Compared to DevOps, MLOps is more experimental in nature. This new framework requires data scientists to try a variety of features, parameters, and models.
MLOps vs AIOps
It is obvious from the two definitions above that the two domains are different and certainly not to be confused. Even if both share this willingness to make our systems better and more efficient, the two fields overlap but do not meet under the same umbrella, neither in terms of the approach nor in terms of the raison d'être.
While MLOps' goal is bridging the gap between data scientists and operation teams, therefore between ML model building and their execution, AIOps focuses on automating incidents management and intelligent root cause analysis.
Get similar stories in your inbox weekly, for free
Share this story:
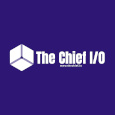
The Chief I/O
The team behind this website. We help IT leaders, decision-makers and IT professionals understand topics like Distributed Computing, AIOps & Cloud Native
Latest stories
How ManageEngine Applications Manager Can Help Overcome Challenges In Kubernetes Monitoring
We tested ManageEngine Applications Manager to monitor different Kubernetes clusters. This post shares our review …
AIOps with Site24x7: Maximizing Efficiency at an Affordable Cost
In this post we'll dive deep into integrating AIOps in your business suing Site24x7 to …
A Review of Zoho ManageEngine
Zoho Corp., formerly known as AdventNet Inc., has established itself as a major player in …
Should I learn Java in 2023? A Practical Guide
Java is one of the most widely used programming languages in the world. It has …
The fastest way to ramp up on DevOps
You probably have been thinking of moving to DevOps or learning DevOps as a beginner. …
Why You Need a Blockchain Node Provider
In this article, we briefly cover the concept of blockchain nodes provider and explain why …
Top 5 Virtual desktop Provides in 2022
Here are the top 5 virtual desktop providers who offer a range of benefits such …
Why Your Business Should Connect Directly To Your Cloud
Today, companies make the most use of cloud technology regardless of their size and sector. …
7 Must-Watch DevSecOps Videos
Security is a crucial part of application development and DevSecOps makes it easy and continuous.The …