Pandora's Flask: Monitoring a Python web app with Prometheus
in Containerization , Visualization , Monitoring and Observability
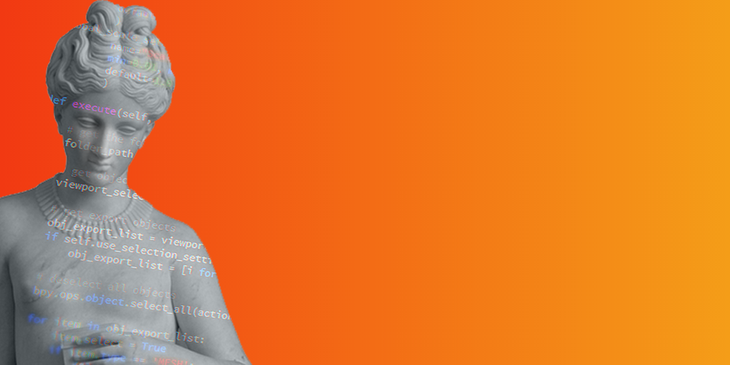
We eat lots of our own dogfood at Metricfire, monitoring our services with a dedicated cluster running the same software.
This has worked out really well for us over the years: as our own customer, we quickly spot issues in our various ingestion, storage and rendering services. It also drives the service status transparency our customers love.
Recently we’ve been working on a new Prometheus offering. Eating the right dogfood here means integrating Prometheus monitoring with our (mostly Python) backend stack.
This post describes how we’ve done that in one instance, with a fully worked example of monitoring a simple Flask application running under uWSGI + nginx. We’ll also discuss why it remains surprisingly involved to get this right.
A little history
Prometheus' ancestor and main inspiration is Google's Borgmon.
In its native environment, Borgmon relies on ubiquitous and straightforward service discovery: monitored services are managed by Borg, so it’s easy to find e.g. all jobs running on a cluster for a particular user; or for more complex deployments, all sub-tasks that together make up a job.
Each of these might become a single target for Borgmon to scrape data from via /varz
endpoints, analogous to Prometheus’ /metrics. Each is typically a multi-threaded server written in C++, Java, Go, or (less commonly) Python.
Prometheus inherits many of Borgmon's assumptions about its environment. In particular, client libraries assume that metrics come from various libraries and subsystems, in multiple threads of execution, running in a shared address space. On the server side, Prometheus assumes that one target is one (probably) multi-threaded program.
Why did it have to be snakes?
These assumptions break in many non-Google deployments, particularly in the Python world. Here it is common (e.g. using Django or Flask) to run under a WSGI application server that spreads requests across multiple workers, each of which is a process rather than a thread.
In a naive deployment of the Prometheus Python client for a Flask app running under uWSGI, each request from the Prometheus server to /metrics
can hit a different worker process, each of which exports its own counters, histograms, etc. The resulting monitoring data is garbage.
For example, each scrape of a specific counter will return the value for one worker rather than the whole job: the value jumps all over the place and tells you nothing useful about the application as a whole.
A solution
Amit Saha discusses the same problems and various solutions in a detailed writeup. We follow option #2: the Prometheus Python client includes a multiprocess mode intended to handle this situation, with gunicorn being the motivating example of an application server.
This works by sharing a directory of mmap()'d dictionaries across all the processes in an application. Each process then does the maths to return a shared view of the whole application's metrics when it is scraped by Prometheus.
This has some "headline" disadvantages listed in the docs: no per-process Python metrics for free, lack of full support for certain metric types, a slightly complicated Gauge type, etc.
It's also difficult to configure end-to-end. Here's what's necessary & how we achieved each part in our environment; hopefully this full example will help anyone doing similar work in the future.
- The shared directory must be passed to the process as an environment variable,
prometheus_multiproc_dir
.
No problem: we use uWSGI's env option to pass it in: see uwsgi.ini. - The client’s shared directory must be cleared across application restarts.
This was a little tricky to figure out. We use one of uWSGI's hardcoded hooks, exec-asap, to exec a shell script right after reading the configuration file and before doing anything else. See uwsgi.ini.
Our script removes & recreates the Prometheus client's shared data directory.
In order to be sure of the right permissions, we run uwsgi under supervisor as root and drop privs within uwsgi. - The application must set up the Python client’s multiprocess mode.
This is mostly a matter of following the docs, which we did via Saha's post: see metrics.py.
Note that this includes some neat middleware exporting Prometheus metrics for response status and latency. - uWSGI must set up the application environment so that applications load after fork().
By default, uWSGI attempts to save memory by loading the application and thenfork()'ing. This indeed has copy-on-write advantages and might save a significant amount of memory.
However, it appears to interfere with the operation of the client's multiprocess mode - possibly because there's some locking prior to fork() this way?
uWSGI's lazy-apps option allows us to load the application after forking, which gives us a cleaner environment.
So altogether, this results in a working /metrics
endpoint for our Flask app running under uWSGI. You can try out the full worked example in our pandoras_flask demo.
Note that in our demo we expose the metrics endpoint on a different port to the app proper - this makes it easy to allow access for our monitoring without users being able to hit it.
In our deployments, we also use the uwsgi_exporter to get more stats out of uWSGI itself.
Futures
Saha's blog post lays out a series of alternatives, with pushing metrics via a local statsd as the favoured solution. That’s not really a hop we prefer to take.
Ultimately, running everything under container orchestration like kubernetes would provide the native environment in which Prometheus shines, but that’s a big step just to get its other advantages in an existing Python application stack.
Probably the most Promethean intermediate step is to register each sub-process separately as a scraping target. This is the approach taken by django-prometheus, though the suggested “port range” approach is a bit hacky.
In our environment, we could (and may yet) implement this idea with something like:
- Running a webserver inside a thread in each process, listening on an ephemeral port and serving /
metrics
queries; - Having the webserver register and regularly refresh its address (e.g. hostname:32769) in a short-TTL etcd path—we use etcd already for most of our service discovery needs;
- Using file-based service discovery in Prometheus to locate these targets and scrape them as individuals.
We think this approach is less involved than using the Python client’s multiprocess mode, but it comes with its own complexities.
It’s worth noting that having one target per worker contributes to something of a time series explosion. For example, in this case a single default Histogram metric to track response times from the Python client across 8 workers would produce around 140 individual time series, before multiplying by other labels we might include. That’s not a problem for Prometheus to handle, but it does add up (or likely, multiply) as you scale, so be careful!
Conclusion
For now, exporting metrics to Prometheus from a standard Python web app stack is a bit involved no matter which road you take. We hope this post will help people who just want to get going with their existing nginx + uwsgi + Flask
apps.
As we run more services under container orchestration—something we intend to do—we expect it will become easier to integrate Prometheus monitoring with them.
Established Prometheus users might like to look at our Hosted Prometheus offering—if you’d like a demo, please get in touch!
Get similar stories in your inbox weekly, for free
Share this story:
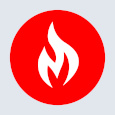
MetricFire
MetricFire provides a complete infrastructure and application monitoring platform from a suite of open source monitoring tools. Depending on your setup, choose Hosted Prometheus or Graphite and view your metrics on beautiful Grafana dashboards in real-time.
Latest stories
How ManageEngine Applications Manager Can Help Overcome Challenges In Kubernetes Monitoring
We tested ManageEngine Applications Manager to monitor different Kubernetes clusters. This post shares our review …
AIOps with Site24x7: Maximizing Efficiency at an Affordable Cost
In this post we'll dive deep into integrating AIOps in your business suing Site24x7 to …
A Review of Zoho ManageEngine
Zoho Corp., formerly known as AdventNet Inc., has established itself as a major player in …
Should I learn Java in 2023? A Practical Guide
Java is one of the most widely used programming languages in the world. It has …
The fastest way to ramp up on DevOps
You probably have been thinking of moving to DevOps or learning DevOps as a beginner. …
Why You Need a Blockchain Node Provider
In this article, we briefly cover the concept of blockchain nodes provider and explain why …
Top 5 Virtual desktop Provides in 2022
Here are the top 5 virtual desktop providers who offer a range of benefits such …
Why Your Business Should Connect Directly To Your Cloud
Today, companies make the most use of cloud technology regardless of their size and sector. …
7 Must-Watch DevSecOps Videos
Security is a crucial part of application development and DevSecOps makes it easy and continuous.The …