Everything You Need To Know About AIOps
in AIOps
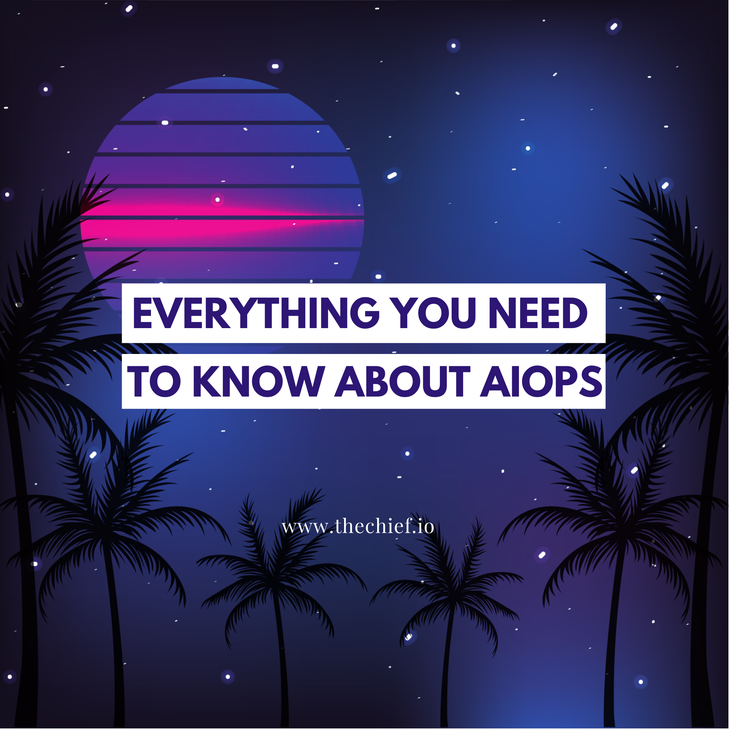
This blog takes you on a deep dive into artificial intelligence and its application in IT operations, AIOps. It explains the concept of AIOps, the goal, use cases, and future prediction in simple concise words to help navigate your journey to an automated and intelligent IT operations workflow.
Thanks to sci-fi movies and animations, when you hear the word "AI," what you think of is a robot with so much power to make your life easier. But, the truth is, if you've ever browsed through Netflix movie suggestions or asked Siri or Alexa for the nearest service station, then you've interacted with artificial intelligence subconsciously.
And that's the point with AI; it is designed to feel less than machine interaction and more like an intelligence similar to humans.
Take a typical chatbot used by small enterprises, for example; it is programmed to reply to predefined message queries and answer your queries with predefined messages or FAQs.
On the other hand, AI is trained to learn from a vast amount of data over time and then develop an algorithm that will help it analyze and solve problems like humans.
Unilever, one of the largest consumer goods companies globally with over a hundred and fifty-three thousand employees across the globe, uses artificial intelligence to make its hiring process more efficient.
Unilever recruits around thirty thousand people a year and, on average, gets over 1.8million applications for this recruitment process. Processing this massive number of applications, sorting, and interviewing the individuals is a huge task that requires a significant amount of time and resources.
To test the efficiency of artificial intelligence technology, the company partnered with Pymetrics. It used AI in its "Future Leaders Programme" recruitment process — a program available for just 800 people but got over two hundred and fifty thousand applications.
Pymetrics designed an online game that first assesses a candidate's reasoning, aptitude, logic, and risk appetite. This is then followed by a second process that requires the candidate to submit a video interview. Of course, this video interview is assessed by artificial intelligence. It uses a combination of machine learning, natural language processing, and body language analysis, which are subsets of artificial intelligence, to assess each candidate and match their profiles against those of previously successful employees.
The interviewing system is also designed to give feedback to all participants with details of their assessment process, whether or not they are suitable for the role.
Like it's used in the streamlining recruitment process as in the case of Unilever, artificial intelligence is also used for predicting flight delays in the aviation industry, diagnosing cancer in the health industry, improving the learning experience in the education industry, and different other use cases across various industries. With the birth of AIOps, IT operations are not left out in this technological revolution.
What is AIOps?
Artificial intelligence for operations or Algorithmic Operations, AIOps, is a term coined by Gartner, an IT research firm, to describe the use of artificial intelligence and machine learning in IT operations.
Artificial intelligence or machine learning in IT operations uses massive data sets to train machines to perform iterative operations tasks in semi-supervised ways. Continuous integration, deployment, delivery, and monitoring are examples of such tasks.
AIOps vs. MLOps: What's the difference?
As much as their difference seems complicated, both terms are distinctive in practice. For example, AIOps and MLOps are two terms that describe the use of artificial intelligence and machine learning but in different cases.
Here is a simple explanation of the difference between AIOps and MLOps;
AIOps refers to the application of machine learning and artificial intelligence to IT operations to streamline repetitive tasks. MLOps, on the other hand, refers to the application of IT operations and DevOps practices in machine learning workflows.
Brief History Of AIOps
Even though it is a relatively new term still gaining momentum among enterprises, machine learning in IT operations can be dated back to 2001, when machine learning emerged in IT operations.
During this period, machine learning is used in operational analytics platforms to perform high-level pattern recognition.
When enterprises began to move to the cloud in 2010, machine learning also experienced more growth in IT operations, increasing the adoption of Big Data to drive business growth.
In 2016, artificial intelligence in IT operations experienced the Gartner effect.
Gartner coined the word “AIOps” in 2016; to describe the lineal usage of machine learning and artificial intelligence to improve the IT operations workflow.
It also defined the aims and benefits of AIOps, some of which we will elaborate on later in this blog.
Gartner also predicted that by 2019, 25% of global enterprises would have strategically implemented an AIOps platform supporting two or more major IT operations functions.
Since then, AIOps has experienced massive growth as cloud technology and IT operations evolve.
The Goal of AIOps
The goal of applying artificial intelligence into IT operations is to automate IT processes, intelligently collect and analyze data, and keenly resolve IT issues.
AIOps also helps bring IT monitoring, visibility, and management together to realize a continuous insight that helps to provide lasting solutions and improvements to IT issues.
To achieve this goal, AIOps combines the three disciplines in IT operations; service management, performance management, and automation.
In 2019, a senior Director Analyst at Gartner, Padraig Bryne, said, "IT operations are challenged by the rapid growth in data volumes generated by IT infrastructure and applications that must be captured, analyzed and acted on." This sentence summarises the goal of AIOps.
Benefits of AIOps
An outline of the benefits of artificial intelligence in IT operations is that; AIOps reduces cost and increases revenue.
In addition to that, below are some of the benefits of adopting AIOps in your organization.
Automation
Probably the most exciting part of AIOps technology is automation. From the simple to the more complicated operations tasks, AIOps allows you to automate or partially automate major challenging tasks, leaving you only to supervise the activities.
Improves Monitoring
With IT operations generating thousands of data every minute, monitoring your system environment and collecting data for analytical usage is problematic. AIOps eases this burden by providing insightful data visualization through AIOps platforms.
Enhances Productivity
Leveraging the potential of AIOps improves the efficiency and productivity of the IT operations team.
IT operations involve many repetitive and challenging tasks, such as the collection of data and environmental monitoring. Leveraging the automation offered by AIOps will help IT operations professionals simplify and automate their repetitive tasks, enabling them to work faster and focus on more business and performance-oriented tasks.
Cost Savings
An essential factor to consider when thinking of any cloud technology is cost saving. Operating in the cloud can be costly when not done right, but AIOps clears you of such cases. In addition, AIOps gives significant savings on operational costs since many operations tasks are done automatically rather than manually. As a result, the IT team has fewer tasks on their hands.
Improved Customer Experience
AIOps helps your organization build and manage business solutions efficiently. Artificial intelligence helps you diagnose and identify potential performance glitches in your application and gives you recommendations to help you resolve them before your customers notice them. This improves the overall user experience of your applications as users experience less disruption and more efficiency using your application.
AIOps also helps enterprises address complex IT challenges, like; dynamicity of IT architectures, digital business transformation, siloed IT operations.
Use Cases of AIOps
Even though in what case AIOps is used differs from one enterprise to another, below are some of the typical use cases of AIOps in an IT environment.
Anomaly detection
DevOps teams often detect faults and anomalies in their infrastructure when a user reports a horrible service experience. This requires them to quickly identify the problem, find and fix the bug manually. But with the complex data systems in modern systems, the task gets too complicated and less effective. AIOps leverage artificial intelligence, machine learning, and deep learning to compare real-time performance metrics with past analytics to identify and detect system anomalies.
Root cause analysis
Rather than just solving it on the surface, it is crucial to find the underlying cause of an issue is in the first place. Therefore, in addition to detecting anomalies, AIOps can collect and correlate events then use machine learning inference models in segregating related events to identify the root cause of issues.
IT noise reduction
By "IT noise," we mean false or unnecessary alerts and notifications that hinder IT personnel from figuring out the real issue with the system and resulting in a waste of time and resources. Artificial intelligence and machine learning algorithms solve this problem by automatically collecting and correlating, and calculating alerts across the application stacks. This ensures that only data that IT teams can leverage to identify root causes of anomalies are alerted, saving them time and energy used in manual analysis.
Event Correlation
According to an AIOps exchange report, 40% of companies get over 1 million incident alerts in a day. Unfortunately, that results in alert fatigue, leading IT professionals to ignore critical warnings that may cause system downtime.
AIOps collects these alerts, analyzes them to find relationships between the data, and groups them into a smaller number of notifications, ensuring only issues with high business value are alerted.
What are AIOps Platforms?
An AIOps platform collects data from various sources, ingests it, and uses artificial intelligence to sort, process the data, and give visibility and recommendations for an IT environment.
AIOps platforms utilize different aspects of artificial intelligence such as machine learning and big data, collect data from various application sources, and intelligently spot, react, and alarm IT issues in real-time.
An AIOps platform plays the multiple roles of a typical data collection, organization, monitoring, and management tools, thereby helping IT teams work faster and more efficiently from a unified interface.
Some examples of AIOps platforms are;
- PagerDuty
- Datadog
- Dynatrace
- Moogsoft
- BigPanda
- AppDynamics
- Loglizer
- Seldon Core
The AIOps Market
Growing from USD 1.73 billion in 2017 to USD 13.51 billion in 2020, the AIOps market experienced a growth of 87% within three years.
A 2021 research by Mordor Intelligence also predicts that the market will experience further 21.05% growth between 2021 and 2026, reaching up to USD 40.91 billion in market size.
This massive growth and highly optimistic prediction are a result of the increasing adoption of the technology.
With its biggest market in Asia and Europe, AIOps' prominent market players include Moogsoft, Dynatrace, Splunk, BMC Software, and Sumo Logic.
Since 2017, Gartner has released an annual report named “Market Guide for AIOps Platforms.” This report provides findings from Gartner's research into the latest development in the AIOps industry and analyses of the previous year.
The report contains a response to questions like why organizations need a single, domain agnostic AIOps platform, why enterprises are adopting AIOps platforms to supplement traditional monitoring tools, how to evaluate whether or not your organization should consider adopting AIOps, which IT use cases AIOps suits and why AIOps vendors need to focus on domain-agnostic AIOps solutions.
AIOps Open source vs AIOps SaaS
Understanding the criteria of a good AIOps platform, types of AIOps offerings, and the difference between an open source AIOps solution and a software-as-a-service (SaaS) AIOps solution is an excellent way to determine which one to use in your organization.
Criteria of a good AIOps platform
For any software, SaaS or open source, to be recommended as an AIOps platform, it needs to entail the following features.
Data Ingestion
An AIOps platform must be able to ingest data from various sources. This is a crucial feature of any AIOps platform because every AIOps platform's functions begin with data.
Artificial Intelligence
As the name AIOps denotes, what is an AIOps tool without the ability to deploy and exhibit artificial intelligence?
Artificial intelligence helps an AIOps platform process the data ingested in the first step and use other sub-technologies such as pattern recognition and machine learning to sort the data and provide intelligent visibility and recommendation.“Moogsoft AIOps and HCL’
Automation
Another crucial feature of an AIOps platform is the ability to automate tasks. It should be able to automate tasks such as analysis, data visualization, monitoring, and alerting.
Types of AIOps Offerings
Gartner grouped AIOps platforms in two offering types; domain-agnostic and domain-centric.
Domain-centric AIOps platforms tend to have limitations to their flexibility. Because it revolves around a specific network domain and endpoint, it is restricted to particular data types and sources.
Examples of domain-centric AIOps platforms include Dynatrace, AppDynamics, and Datadog.
Domain-agnostic AIOps platforms, on the other hand, have the flexibility to ingest a large volume of data from various sources, thereby making it useful for multiple use cases.
Examples of domain-agnostic AIOps platforms include BigPanda, Moogsoft, and Splunk.
Difference between Open source AIOps and SaaS AIOps platforms
A primary and distinctive difference between an open source AIOps tool and a SaaS tool is that; open source AIOps tools are usually free, customizable, and pose less risk of getting stuck to a vendor.
A downside of open source AIOps platforms is that you cannot get an end-to-end AIOps solution with one platform unless you combine two or more. This is why open source AIOps platforms are preferably referred to as AIOps tools.
In contrast, SaaS AIOps platforms are proprietary software. They offer a full-featured, managed AIOps solution which can guarantee security compliance and mitigate the risks of data misuse.
All these, however, come at a price.
AIOps Case Study
A typical case study of AIOps in action is the case of HCL Technologies, an international managed IT services company.
As an IT-based company, HCL was faced with challenges such as operational noise, alert fatigue, long root cause analysis, and service disruption.
At the time, the company used a traditional rule-based filtering and correlation approach to manage IT issues. However, as the complexity of their IT environment increases and the partial move to the cloud, HCL experienced a surge in events and costs.
“The move to agile and cloud increases the raw events and log rates exponentially, overwhelming operational support staff,” the CTO of HCL technologies lamented.
To solve this problem, HCL Technologies consulted a popular AIOps vendor, Moogsoft.
On implementing the Moogsoft solution, HCL experienced a 62% reduction in event tickets, seamless platform migration, and significant cost savings, to mention a few.
Why AIOps is the future
There is a popular phrase that goes: "it can only get better."
Especially in technologies that offer as much AIOps does, more is to be expected.
In the coming, AIOps is expected to experience an increase in adoption and eventually become a de facto technology in IT operations.
In Gartner's Innovation Insight for Observability report, it predicted that 40% of DevOps teams will augment application and infrastructure monitoring tools with AIOps platform capabilities”
Following the optimistic market size prediction by Mordor Intelligence, Gartner's prediction signals that AIOps will continue to dominate the IT workflows now and in the future.
Required Skill Sets To Deploy AIOps
With the adoption and impact of AIOps in the IT industry, IT professionals need to adapt to certain skill sets in order to evolve with the technology.
In addition to the standard IT skills, professionals dealing with AIOps need to be equipped with monitoring and auditing skills.
Artificial intelligence is a "machine at work" and it requires constant supervision to make the most out of it.
Hence, IT professionals willing to utilize AIOps need to upgrade their skillsets to be able to audit and manage machine learning processes efficiently.
Conclusion
AIOps introduces the intelligence needed to manage the complexity of modern IT environments. In addition to all you’ve read in this blog, you need to understand how to evaluate your IT environment and determine whether or not you are ready to adopt AIOps in your workflows. The State of AIOps: What Do Companies Need to Adopt AIOps? and Gartner’s Market Guide for AIOps Platforms are great resources that will help you start your AIOps journey on the right foot.
Get similar stories in your inbox weekly, for free
Share this story:
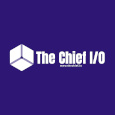
The Chief I/O
The team behind this website. We help IT leaders, decision-makers and IT professionals understand topics like Distributed Computing, AIOps & Cloud Native
Latest stories
How ManageEngine Applications Manager Can Help Overcome Challenges In Kubernetes Monitoring
We tested ManageEngine Applications Manager to monitor different Kubernetes clusters. This post shares our review …
AIOps with Site24x7: Maximizing Efficiency at an Affordable Cost
In this post we'll dive deep into integrating AIOps in your business suing Site24x7 to …
A Review of Zoho ManageEngine
Zoho Corp., formerly known as AdventNet Inc., has established itself as a major player in …
Should I learn Java in 2023? A Practical Guide
Java is one of the most widely used programming languages in the world. It has …
The fastest way to ramp up on DevOps
You probably have been thinking of moving to DevOps or learning DevOps as a beginner. …
Why You Need a Blockchain Node Provider
In this article, we briefly cover the concept of blockchain nodes provider and explain why …
Top 5 Virtual desktop Provides in 2022
Here are the top 5 virtual desktop providers who offer a range of benefits such …
Why Your Business Should Connect Directly To Your Cloud
Today, companies make the most use of cloud technology regardless of their size and sector. …
7 Must-Watch DevSecOps Videos
Security is a crucial part of application development and DevSecOps makes it easy and continuous.The …